基于感兴趣区域的图像检索技术
来源:56doc.com 资料编号:5D26567 资料等级:★★★★★ %E8%B5%84%E6%96%99%E7%BC%96%E5%8F%B7%EF%BC%9A5D26567
资料以网页介绍的为准,下载后不会有水印.资料仅供学习参考之用. 密 保 惠 帮助
资料介绍
基于感兴趣区域的图像检索技术(论文11000字,参考代码)
摘要:在信息技术高速发展的时代,传统的基于文本的图像检索技术,已经难以适应庞大的图像数据,基于内容的图像检索技术成为研究的热点。在图像内容中,背景图像的冗余信息,很大程度影响图像检索的准确度,针对这一问题,提出了一种基于感兴趣区域的图像检索技术。先通过边缘检测,检测图像主体轮廓,再通过形态学处理,提取出感兴趣区域,并提取HSV颜色特征Tamura纹理特征融合,作为特征向量。最后,计算融合特征间的标准欧氏距离,并定义距离近的图像为相似图像。本文在Corel-1000图像数据集上进行图像检索实验,实验表明,该方法所提取的感兴趣区域与人类视觉较一致,并且在图像检索方面,有较高的查准率与查全率。
关键词:感兴趣区域;多特征融合;图像检索;Tamura;HSV
Image Retrieval Technology Based on Region of Interest
Abstract:In the rapid development of information technology, the traditional text based image retrieval technology has been difficult to adapt to the huge image data, and the content based image retrieval technology has become a hot spot of research. In the image content, the redundant information of the background image greatly affects the accuracy of the image retrieval. In view of this problem, an image retrieval technique based on the region of interest is proposed. First, the edge detection is used to detect the main contour of the image, and then the region of interest is extracted by morphological processing, and the HSV color feature Tamura texture feature fusion is extracted as the feature vector. Finally, the standard Euclidean distance between the fused features is computed, and the images near the distance are defined as similar images. The experiment of image retrieval on Corel-1000 image data set shows that the region of interest extracted by this method is more consistent with human vision, and has high precision and recall rate in image retrieval.
Keywords: Region of interest; Multi-feature fusion; Image retrieval; Tamura; HSV
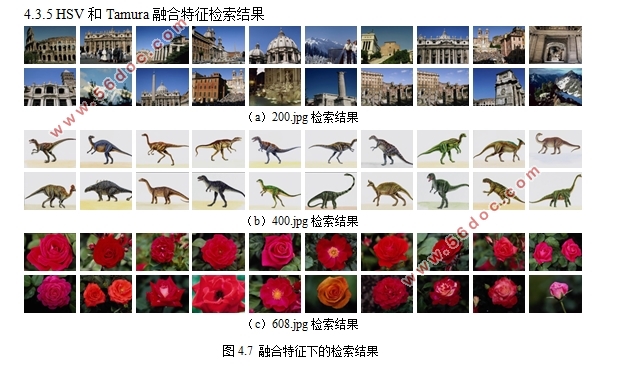
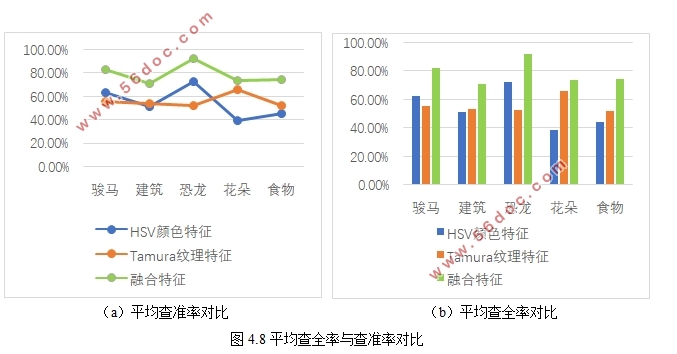
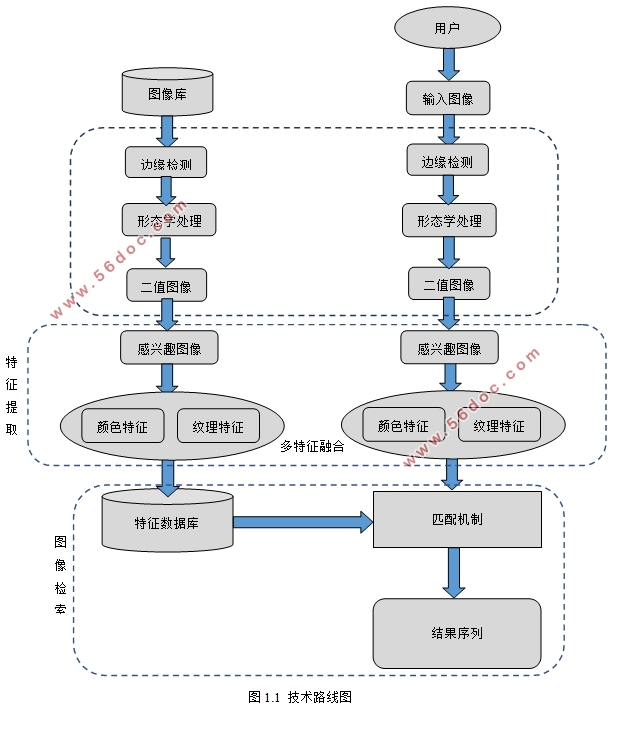
目录
摘要 I
Abstract II
一、引言 1
1.1 研究背景及意义 1
1.2 本文主要研究内容及技术路线 1
1.2.1主要研究内容 1
1.2.2技术路线 1
二、相关技术研究 3
2.1 图像处理的目的 3
2.2 数字图像相关知识 3
2.2.1 数字图像的数学模型 3
2.2.2 图像分辨率 3
2.2.3 数字图像的存储方式 4
2.3 边缘检测技术 5
2.3.1 概述 5
2.3.2坎尼边缘检测 5
2.4 形态学处理技术 7
2.4.1 形态学处理的目的 7
2.4.2 形态学处理常用技术 8
2.5 图像特征提取 9
2.5.1 颜色特征 9
2.5.1纹理特征 10
三、感兴趣区域提取及多特征融合的图像检索算法 12
3.1 算法流程 12
3.2 图像预处理 13
3.3 特征融合与相似性度量算法 13
3.4 性能评估方法 14
四、实验及结果分析 16
4.1 实验环境设置 16
4.2 实验数据集 16
4.3 实验结果 17
4.3.1 Canny边缘提取结果 17
4.3.2 感兴趣区域提取结果 17
4.3.3 HSV特征检索结果 17
4.3.4 Tamura特征检索结果 18
4.3.5 HSV和Tamura融合特征检索结果 19
4.4 分析 20
4.4.1 HSV、Tamura特征的查准率、查全率和平均检索时间 20
4.4.2 融合特征在不同权值下的查准率、查全率和平均检索时间 21
4.4.3 对比分析 21
五、总结与展望 22
参考文献 23
致谢 23
|